Deep Photometric Stereo
A deep learning enabled photometric stereo method for confidence-aware surface height and normal reconstruction.
Three-dimensional (3D) measurement provides essential geometric information for quality control and process monitoring in many manufacturing applications. Photometric stereo is one of the potential solutions for in-process metrology and active geometry compensation, which takes multiple images of an object under different illuminations as inputs and recovers its surface normal map based on a reflectance model.
Deep learning approaches have shown their potential in solving the highly nonlinear problem for photometric stereo, but the main challenge preventing their practical application in process metrology lies in the difficulties in the generation of a comprehensive dataset for training the deep learning model.
This project proposes a new Deep-learning based Point-light Photometric Stereo method, DPPS, which utilizes a multi-channel deep convolutional neural network (CNN) to achieve end-to-end prediction for both the surface normal and height maps in a semi-calibrated fashion (Yang et al., 2023). The key contribution is a new dataset generation method combining both physics-based and data-driven approaches, which minimizes the training cost and enables DPPS to handle reflective metal surfaces with unknown surface roughness.
We propsoed a new coarse-to-fine network to improve the performance by exploiting the relationship between initial normal and depth predictions. Furthermore, the pixel-wise confidence associated with predictions is also estimated without requiring the ground truth, making a contribution to enhancing both performance and practicality. The experimental results on our synthetic dataset and real samples demonstrate the effectiveness of the proposed method on both normal/depth and confidence estimation (Zhang et al., 2025)
Codes are released on GitHub.
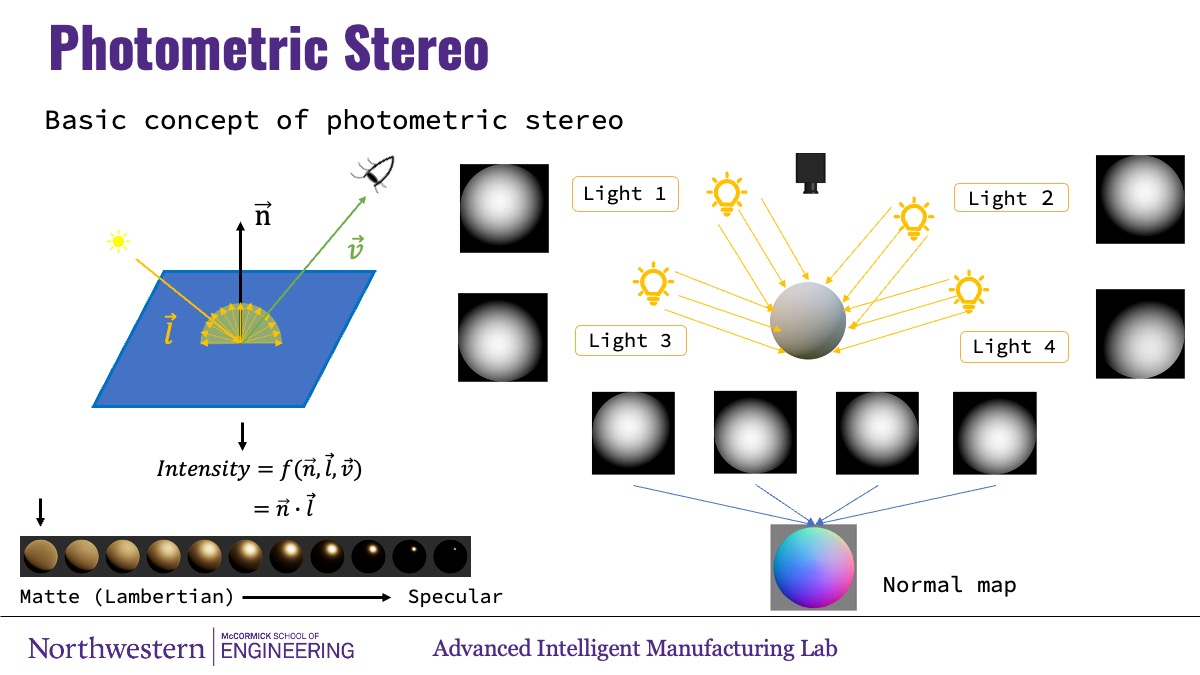
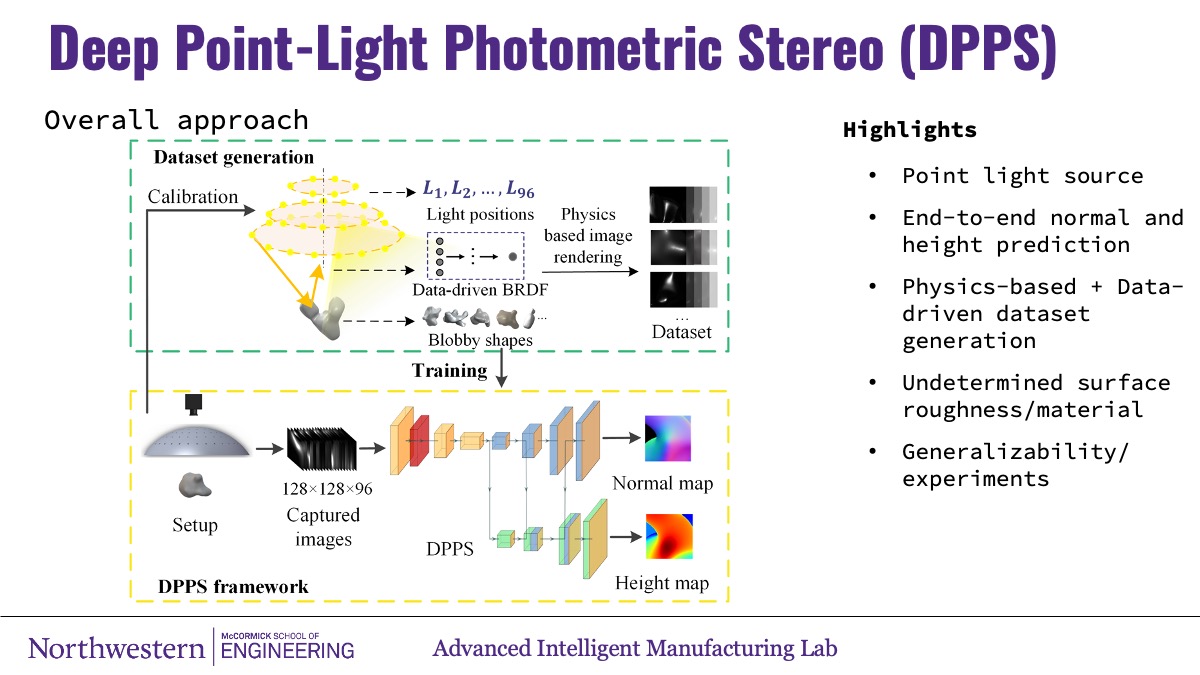
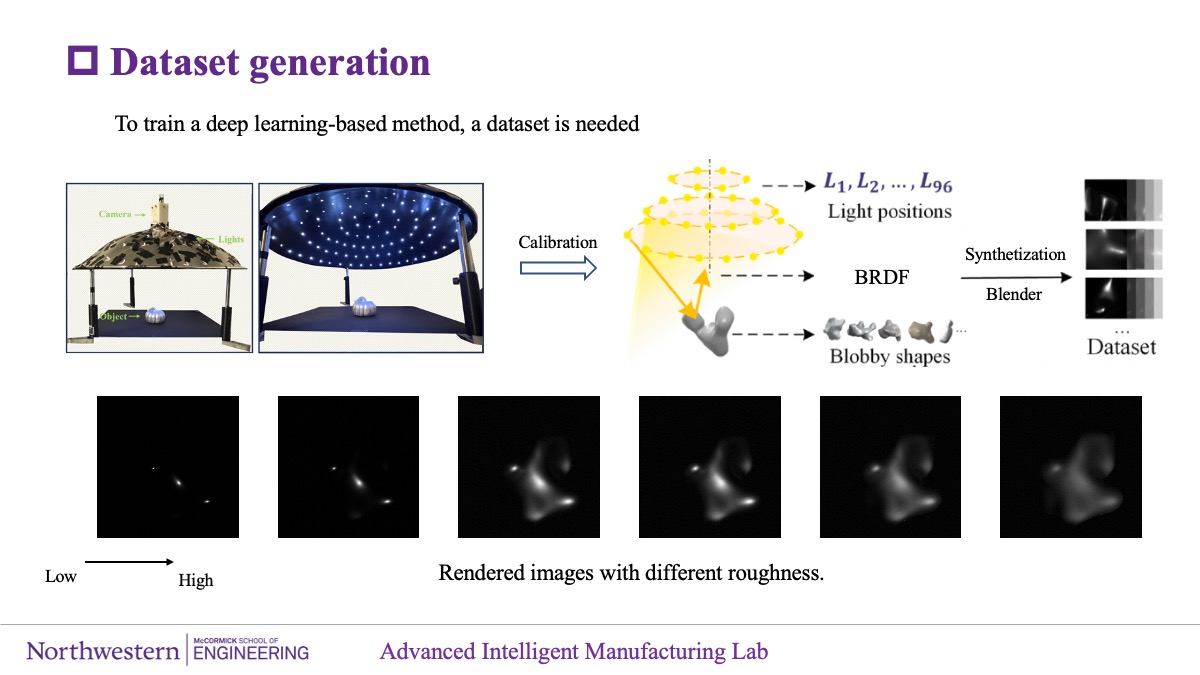
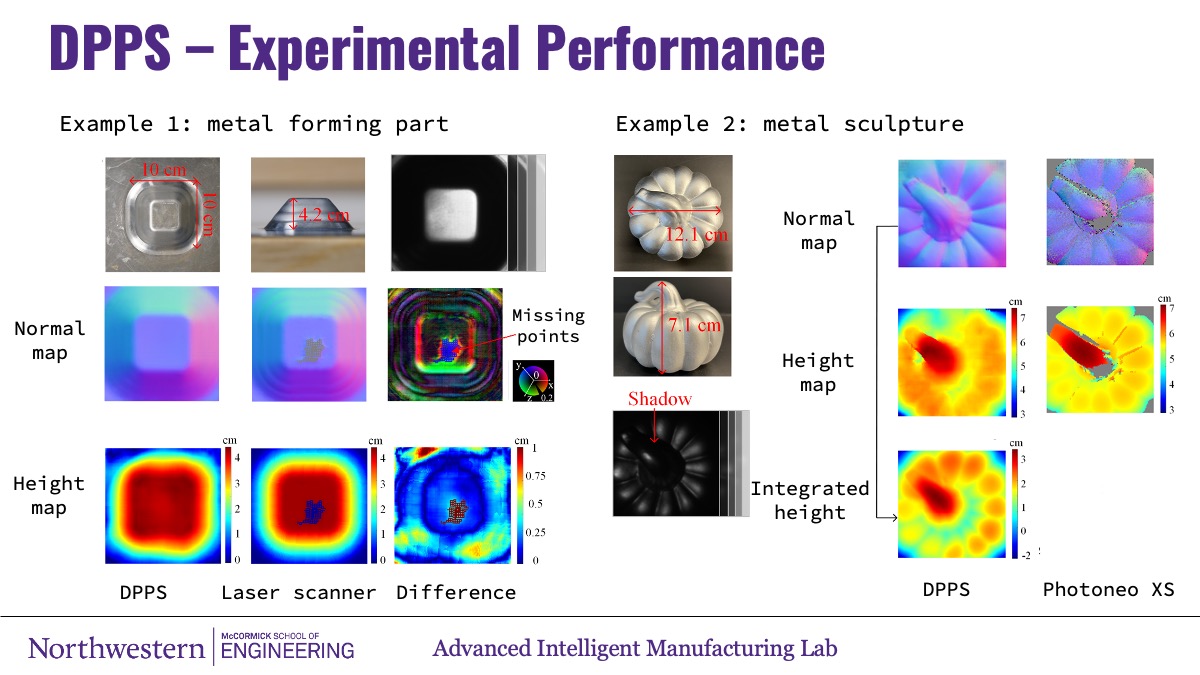
References
2025
-
IEEE/ASME Transactions on Mechatronics, 2025